AI - Population Prediction
Predicting the future isn’t magic—it’s data. Our AI-Based Population Prediction Model helps governments, businesses, and organizations make smarter decisions by forecasting population trends with accuracy. Whether it’s planning better cities, preparing for healthcare demands, or understanding where new businesses should open, our model analyzes real-world factors like birth rates, migration, and urbanization. By combining machine learning, deep learning, and statistical forecasting, we turn raw data into meaningful insights that help you plan for what’s ahead.
Technologies Used
- Python
- Pandas & NumPy
- Matplotlib & Seaborn
- Scikit-Learn
- XGBoost
- Statsmodels
- Prophet (by Facebook)
- LSTMs (Deep Learning)
We know data can feel overwhelming, but we make it simple. Using Python, Scikit-Learn, and powerful forecasting tools, our AI processes massive datasets, cleans up the noise, and delivers clear predictions. Want to know how a city’s population will grow? Or how many schools will be needed in the next decade? Our model gives you real-time, actionable insights to make informed decisions. Whether you’re a business leader, urban planner, or policymaker, we help you stay ahead of the curve and make decisions with confidence.
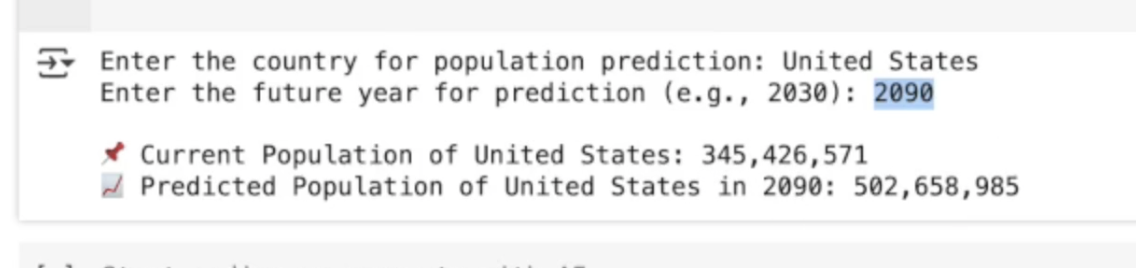
Why Build This AI?
The AI has several real-world uses, such as:
- Mental Health & Well-being Monitoring – Mood tracking apps, therapy support
- Customer Experience & Engagement – Retail satisfaction analysis, chatbot responses
- Education & E-Learning – Student engagement detection in online learning
- Marketing & Advertising – Audience reaction analysis, ad effectiveness testing
- Smart Surveillance & Security – Unusual behavior detection in public spaces
Let's Build It
Data Processing & Model Preparation
- Install Required Libraries → Ensures all dependencies (like Pandas, NumPy, Scikit-Learn, etc.) are available.
- Import Libraries → Loads necessary libraries for data analysis, visualization, and machine learning.
- Data Handling (Pandas & NumPy) → Helps in loading, manipulating, and processing datasets.
- Feature Scaling (StandardScaler) → Standardizes numerical features for better model performance.
- Machine Learning (RandomForestRegressor) → Implements a powerful regression model to predict future populations.
- Model Evaluation (MAE, MSE, R² Score) → Measures the model’s accuracy and performance.
# Install required libraries (if not already installed)
!pip install pandas numpy scikit-learn matplotlib seaborn --quiet
# Import necessary libraries
import pandas as pd
import numpy as np
import matplotlib.pyplot as plt
import seaborn as sns
from sklearn.model_selection import train_test_split
from sklearn.preprocessing import StandardScaler
from sklearn.ensemble import RandomForestRegressor
from sklearn.metrics import mean_absolute_error, mean_squared_error, r2_score
Loading the Dataset in Google Colab
- Upload Dataset → Uses
files.upload()
to let the user select and upload a CSV file. - Read CSV File → Extracts the file name dynamically and loads it into a Pandas DataFrame (
df
). - Display Data Preview → Shows the first few rows of the dataset to verify successful loading.
from google.colab import files
# Upload the dataset in Google Colab
uploaded = files.upload()
# Read the uploaded CSV file
file_name = next(iter(uploaded)) # Get the uploaded file name
df = pd.read_csv(file_name)
# Display first few rows
print("✅ Dataset Loaded Successfully!")
df.head()
Data Cleaning & Preprocessing
- Display Dataset Info (Before Cleaning) → Provides an overview of missing values and data types.
- Convert ‘Yearly Change’ to Decimal → Converts percentages (e.g., "1.2%" → 0.012).
- Clean ‘Urban Pop %’ Column →
- ✔ Removes % signs.
- ✔ Replaces "N.A." values with NaN.
- ✔ Converts to numeric values.
- ✔ Fills missing values with the mean.
- Convert ‘World Share’ to Decimal → Similar conversion as 'Yearly Change' for easier calculations.
- Convert Numerical Columns →
- ✔ Removes commas (,) in large numbers.
- ✔ Ensures correct numeric data types for accurate computation.
- Display Dataset Info (After Cleaning) → Confirms that all values are correctly formatted and no critical data is missing.
# Display dataset info before cleaning
print("\n🔍 Dataset Info (Before Cleaning):")
df.info()
# Convert 'Yearly Change' from percentage to decimal
df["Yearly Change"] = df["Yearly Change"].astype(str).str.replace('%', '').astype(float) / 100
# Clean 'Urban Pop %' (remove '%', replace "N.A." with NaN, convert to float)
df["Urban Pop %"] = df["Urban Pop %"].astype(str).str.replace('%', '').replace("N.A.", np.nan)
df["Urban Pop %"] = pd.to_numeric(df["Urban Pop %"], errors='coerce')
# Fill missing values in 'Urban Pop %' with the mean
df["Urban Pop %"] = df["Urban Pop %"].fillna(df["Urban Pop %"].mean())
# Convert 'World Share' from percentage to decimal
df["World Share"] = df["World Share"].astype(str).str.replace('%', '').astype(float) / 100
# Convert numerical columns by removing commas
columns_to_clean = ["Population (2024)", "Net Change", "Density (P/Km²)", "Land Area (Km²)", "Migrants (net)"]
for col in columns_to_clean:
df[col] = df[col].astype(str).str.replace(',', '')
df[col] = pd.to_numeric(df[col], errors='coerce')
# Display dataset info after cleaning
print("\n✅ Dataset Cleaning Complete!")
df.info()
Feature Selection & Correlation Analysis
- Define Features & Target Variable →
- ✔ Features (
X
): Includes economic and demographic factors like Yearly Change, Net Change, Density, Fertility Rate, Median Age, Urban Population, etc. - ✔ Target (
y
): The predicted value → Population (2024).
- ✔ Features (
- Display Selected Features → Ensures that the correct features are chosen before model training.
- Correlation Heatmap →
- ✔ Helps identify relationships between numerical variables.
- ✔ Uses only numeric columns to avoid errors.
- ✔ Strong correlations help improve model accuracy by identifying relevant features.
# Select features and target variable
features = ["Yearly Change", "Net Change", "Density (P/Km²)", "Land Area (Km²)", "Migrants (net)", "Fert. Rate", "Med. Age", "Urban Pop %"]
target = "Population (2024)"
# Create feature (X) and target (y) datasets
X = df[features]
y = df[target]
# Display selected features
print("\n🎯 Selected Features & Target:")
X.head()
# 📊 Correlation Heatmap (Only Numeric Data)
plt.figure(figsize=(10,6))
# Select only numeric columns for correlation
numeric_df = df.select_dtypes(include=['number'])
sns.heatmap(numeric_df.corr(), annot=True, cmap="coolwarm", fmt=".2f")
plt.title("📊 Feature Correlation Heatmap")
plt.show()
Data Splitting & Scaling
- Split Dataset into Training & Testing Sets →
- ✔ 80% Training Data → Used to train the AI model.
- ✔ 20% Testing Data → Used to evaluate model performance.
- Feature Scaling (Standardization) →
- ✔ Uses
StandardScaler()
to transform data into a standardized range (mean = 0, variance = 1). - ✔ Ensures that all features have equal importance and prevents large values from dominating the learning process.
- ✔ Uses
# Split data into Training (80%) and Testing (20%) sets
X_train, X_test, y_train, y_test = train_test_split(X, y, test_size=0.2, random_state=42)
# Scale the features for better performance
scaler = StandardScaler()
X_train_scaled = scaler.fit_transform(X_train)
X_test_scaled = scaler.transform(X_test)
print("✅ Data Splitting & Scaling Complete!")
AI Model Training & Evaluation
- Train a Random Forest Regressor →
- ✔ Uses 200 decision trees (
n_estimators=200
) for better predictions. - ✔ Limits tree depth (
max_depth=15
) to prevent overfitting.
- ✔ Uses 200 decision trees (
- Make Predictions →
- ✔ Uses the trained model to predict population for test data.
- Model Evaluation →
- ✔ Mean Absolute Error (
MAE
) → Measures average prediction error. - ✔ Mean Squared Error (
MSE
) → Penalizes large errors more heavily. - ✔ R² Score → Measures how well the model explains data variability (closer to 1 is better).
- ✔ Mean Absolute Error (
# Train a Random Forest model
model = RandomForestRegressor(n_estimators=200, max_depth=15, random_state=42)
model.fit(X_train_scaled, y_train)
# Predict on test data
y_pred = model.predict(X_test_scaled)
# Evaluate the model
mae = mean_absolute_error(y_test, y_pred)
mse = mean_squared_error(y_test, y_pred)
r2 = r2_score(y_test, y_pred)
print(f"📊 Model Evaluation:")
print(f"🔹 Mean Absolute Error (MAE): {mae:.2f}")
print(f"🔹 Mean Squared Error (MSE): {mse:.2f}")
print(f"🔹 R² Score: {r2:.4f} (Higher is better)")
User-Driven Population Prediction
- User Input →
- ✔ User enters the country and target year for prediction.
- Data Extraction →
- ✔ Retrieves historical data for the selected country.
- AI Prediction →
- ✔ Uses exponential growth formula based on Yearly Change Rate to estimate future population.
- Output Results →
- ✔ Displays current population and predicted population in the selected year.
# 📌 User selects a country and future year
country_input = input("Enter the country for population prediction: ")
year_input = int(input("Enter the future year for prediction (e.g., 2030): "))
# Find the selected country in the dataset
if country_input in df["Country"].values:
country_data = df[df["Country"] == country_input]
# Extract required features
yearly_change = country_data["Yearly Change"].values[0]
net_change = country_data["Net Change"].values[0]
density = country_data["Density (P/Km²)"].values[0]
land_area = country_data["Land Area (Km²)"].values[0]
migrants = country_data["Migrants (net)"].values[0]
fertility_rate = country_data["Fert. Rate"].values[0]
median_age = country_data["Med. Age"].values[0]
urban_pop = country_data["Urban Pop %"].values[0]
current_population = country_data["Population (2024)"].values[0]
# Calculate expected population using yearly change
predicted_population = current_population * ((1 + yearly_change) ** (year_input - 2024))
print(f"\n📌 Current Population of {country_input}: {current_population:,.0f}")
print(f"📈 Predicted Population of {country_input} in {year_input}: {predicted_population:,.0f}")
else:
print("❌ Country not found in the dataset. Please try again.")